When it comes to thinking about how artificial intelligence works, at some level, all AI projects are primarily data projects. In order to help think about projects from this perspective, we’ve developed a model which breaks down the essential AI aspect of any project into three main phases: 1) Prepare the relevant data, 2) Train the algorithm(s) and 3) Test the trained algorithm(s).
We can compare these phases to an iceberg to demystify the work of artificial intelligence a little bit. Artificial Intelligence techniques like machine learning, deep learning, and natural language processing are not magic, and much of their success, if not most of their success, depends on extensive data preparation. We estimate that the most significant effort (over half) of a successful AI project is time spent preparing the data, assuming an adequate dataset hasn’t been cleaned and prepared previously. Which is a likely assumption if you are using data from your organization on an AI project for the first time. Yet despite this significant effort, the work of preparing the data is work that largely goes unseen, just like how most of an iceberg is under water and unseen. As such, the complexity of this part of the process is not always appreciated, as it isn’t always reflected in the visible results of a project, in the same way that only a relatively small part of the iceberg can be seen from the surface.
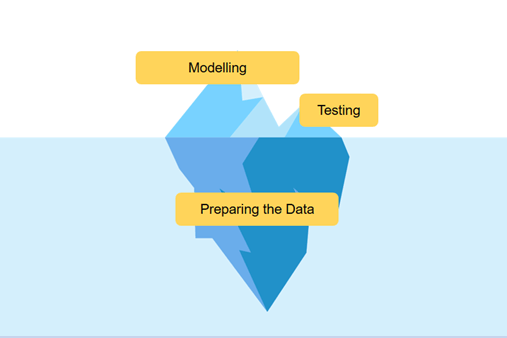
Have you ever used a voice assistant, like Alexa, Siri, or Google Home? Let’s imagine an interaction with Google Home and explore an overview of what happens during each of these phases.
Phase 1) Prepare the Relevant Data
Google Home works by understanding spoken voice commands to take appropriate actions, like answering a question, setting a timer, or controlling some connected device. For these kinds of results to be possible, the first phase, preparing the data, must consist of activities such as:
- collecting millions of voice recordings from a wide range of voices;
- cleaning the sound of the recordings by removing background noise and the like; normalizing the recordings to a single audio format such as mp3;
- labeling the recordings appropriately;
- and other related activities.
Lastly, the data needs to be separated into at least two groups: a group to be used in phase two for modeling the algorithm (training data), and another to be used to test the trained algorithm in phase three (test data).
For an organization like Google, we can imagine that all of the tasks connected to this phase were carried out over the course of years, by a talented team of engineers and developers getting paid to tackle this challenge over the course of multiple iterations and updates. Also, we can imagine that with large technology firms like this, data is their business. They have access to massive amounts of data relevant to compiling robust training data sets and testing data sets for the development of their products. And yet despite all of this, as consumers we’ve probably experienced the imperfections of these devices at one moment or another, where a word or more has been misunderstood or a face has not been recognized.
Now compare those conditions to the resources and data available to any given researcher working in AI, and then we start to understand the magnitude of the data preparation phase and its overall importance in the process of developing a functional AI feature.
Phase 2) Train the Algorithm(s)
Phase two is training the algorithm. First, we choose which algorithm(s) we are going to train. To understand this phase, think of the process of modeling with clay. Imagine that each of these blocks of clay is a different basic algorithm type: the red block represents linear regression, the orange represents k-means, purple represents neural networks, and turquoise represents support vector machines.
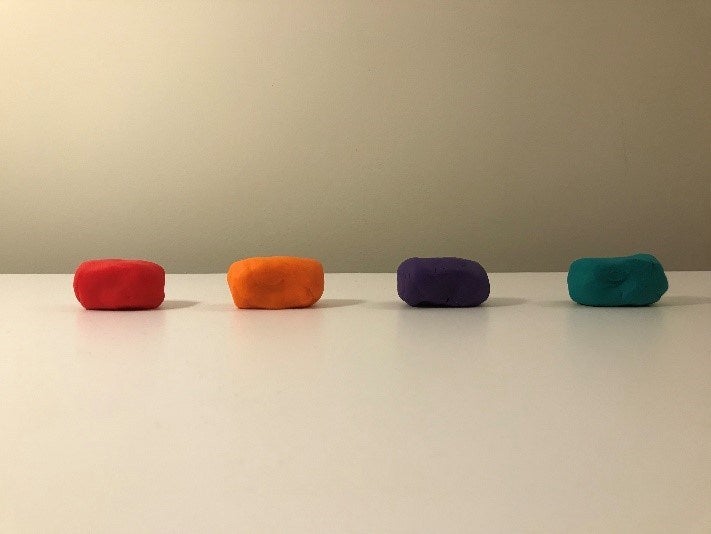
What happens during the training process? You’ve probably heard the expression that you need to find the algorithm that has the best fit. Well, here’s where the clay analogy helps express this idea. During the training process, each algorithm is molded to the training data by finding patterns in the data, so at the end of the phase the algorithms might look like this:
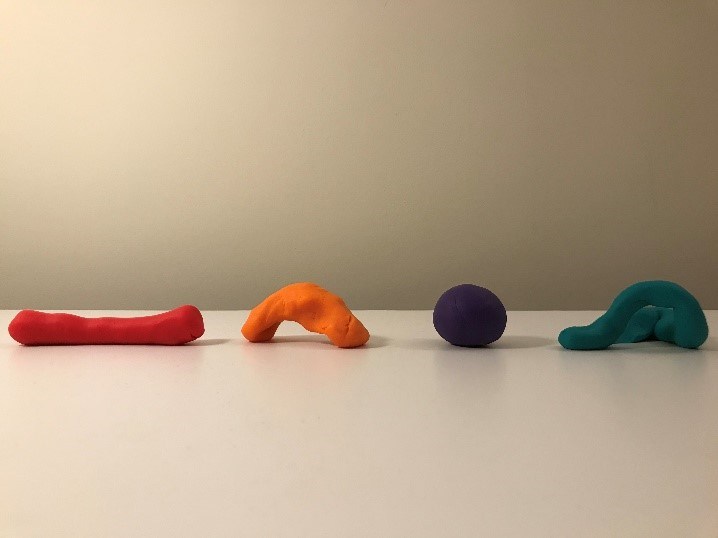
Phase 3) Test the Trained Algorithm(s)
In phase three, testing, each of the trained models is given the testing data to see which one provides the most accurate results and determine how successful our training was. To continue the clay analogy, imagine that a successful test result means being able to roll well. Both the red and the purple trained algorithms in the photo above look like they could roll, but if we test them by sending them down a ramp, the purple trained algorithm will be most effective. In the case of Google Home, if a successful result for a trained algorithm means giving an adequate response to a verbal command based on the testing data, the algorithm that provides the best response would be the best fit.
If the results of the testing phase need improvement, you have essentially two possible next steps: 1) change the algorithms or 2) prepare and introduce additional relevant data. This is an iterative process, but the general sequence follows the three phases of the Iceberg Model.
There are many other considerations when designing and implementing a project that uses artificial intelligence. Our hope is that the Iceberg Model can be a useful conceptual for framing the general approach to your next project as well as communicating the kind of effort which takes place behind the scenes.
What AI projects are you working on?
By Kyle Strand and Daniela Collaguazo from the IDB´s Knowledge, Innovation and Communications Sector
Very good explanation!
Most of the time I hear about AI applications, but never thought of delving deep into the process itself as I thought it was too complicated to be explained to someone with no technical background. Preparing the necessary data turns out to be the most important, yet the least unseen and appreciated. I wonder if this stage (data preparation) could be open to participation by non-technical people since it’s very important to have vast amounts of data for success.
Above article contains more knowledge, Thanks for sharing.
Tanks For Sharing Articel… Good Job